Analysis of a Neural Network Model for Building Energy Hybrid Controls for In-between Season
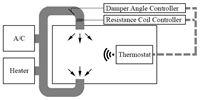
In residential and commercial buildings, programmable thermostats have been practically used to provide appropriate heating and cooling energy to satisfy the thermal conditions. With the help of rapid development of computing technology, recent controllers were able to adopt advanced algorithms such as Fuzzy Inference System (FIS) and Artificial Neural Network (ANN).
The advanced FIS and ANN controllers, which deal with simultaneous control of supply air mass and temperature, are tested to optimize supply air conditions for in-between seasons that require both moderate heating and cooling. The controllers are compared with a thermostat on/off model by means of the total control errors and thermal energy consumption.
The ANN mass and temperature simultaneous control algorithm indicates high efficiency for control errors by 5.59% and effectively mitigates energy increase by 3.95% in comparison with the thermostat on/off controller. Even though the ANN model can effectively reduce control errors for thermal comfort, it consumes less energy than FIS model and a similar amount of energy for a thermostat on/off controller. Under building conditions requiring more sensitive controls and consuming large amounts of energy, the ANN controller can be used to effectively optimize the supply air conditions.
keywords: Building Control, Neural Network Model, Mass & Temperature Control, In-between Season, Energy and Control Efficiency
Add comment
Log in to post comments